浙江大学网络空间安全学院
地点: 浙大玉泉校区曹光彪主楼201
时间: 2023年5月22日 14:00-17:00
01
崔 颖
PhD
Associate Professor
Internet of Things Thrust
Optimal Design for Wireless Communications, Networking, Signal Processing, and Multimedia Transmissions
摘 要
This talk presents recent research in optimization and learning and their applications. The topics include massive machine-type communications (mMTC), intelligent reflecting surface (IRS), mobile edge computing and caching, cross-layer control, distributed/federated learning, immersive video streaming, etc. The results demonstrate the critical roles of optimization and learning in various fields.
报告人简介
Prof. Ying Cui received her B.Eng degree in Electronic and Information Engineering from Xi’an Jiao Tong University, China, in 2007, and her Ph.D. degree from the Hong Kong University of Science and Technology, Hong Kong, in 2012. She held visiting positions at Yale University, US, in 2011 and Macquarie University, Australia, in 2012. From June 2012 to June 2013, she was a postdoctoral research associate at Northeastern University, US. From July 2013 to December 2014, she was a postdoctoral research associate at the Massachusetts Institute of Technology, US. From January 2015 to July 2022, she was an Associate Professor at Shanghai Jiao Tong University, China. Since August 2022, she has been an Associate Professor with the IoT Thrust at The Hong Kong University of Science and Technology (Guangzhou) and an Affiliate Associate Professor with the Department of ECE at The Hong Kong University of Science and Technology. Her current research interests include optimization, learning, IoT communications, mobile edge caching and computing, multimedia transmission, etc. She was selected to the Thousand Talents Plan for Young Professionals of China in 2013. She received Best Paper Awards from IEEE ICC 2015 and IEEE GLOBECOM 2021. She serves as an Editor for the IEEE Transactions on Wireless Communications.
02
Dirk Kutscher
PhD
Associate Professor
Internet of Things Thrust
Computing in the Network: Challenges and Research Opportunities
摘 要
Distributed Machine Learning, social AR/VR and other emerging applications raise the question of how networks can support distributed applications better. This talk discusses the potential and the challenges for "Computing in the Network" – an attempt to integrate computing and networking to create more elastic and resilient infrastructure for applications. We review the state of the art for Computing in the Network and introduce a new research direction called "Compute-First Networking".
报告人简介
Prof. Dr. Dirk Kutscher is a professor at the Hong Kong University of Science and Technology in Guangzhou, China – HKUST(GZ), where is co-directing the Future Networked Systems Laboratory (FNSL). Dirk's main interests lie in the intersection of distributed computing and networking and in Internet architecture. Recently, Dirk has initiated a new research direction called "Compute-First Networking" towards re-imaging the relationship of networking and computing.
Previously he has been a professor for computer science and network at the University of Applied Sciences in Emden/Leer, Germany, the CTO for Virtual Networking and IP at Huawei's German Research Center, the Chief Researcher for Networking at NEC Laboratories Europe, and a Visiting Researcher at KDDI R&D Laboratories in Japan. He is co-chairing two Research Groups in the Internet Research Task Force (IRTF) on Information-Centric Networking (ICNRG) and on Decentralized Internet Infrastructure (DINRG). Dirk has published several IETF RFCs, books, and research publications on Internet technologies. He holds a PhD degree from Universität Bremen, Germany and has been working on making the Internet work better since his PhD study times.
03
巩紫君
PhD
Assistant Professor
Internet of Things Thrust
Information Fusion in Localization
摘 要
For localization, information fusion is not a new topic. However, does information fusion always bring significant performance improvement? In this presentation, we will take the underwater localization of a safe box as an example, and show the power of information fusion in localization. Specifically, we will focus on the search of an underwater target that can constantly broadcast a beacon signal, such as a black box. Common measurements for localization are Doppler shift, time of arrival (ToA), time difference of arrival (TDoA), angle of arrival (AoA) , etc. In this presentation, we will investigate the fundamental limits of Doppler shift-, ToA-, TDoA-based underwater localization. Note that AoA is not covered, because Doppler shift can be viewed as one type of AoA. The discussion will focus on short-baseline positioning with a mobile anchor, i.e., an autonomous underwater vehicle (AUV). Due to the large distance and the limited battery life of the AUV, the target is quite likely to lie outside the convex hull of the AUV's trajectory. In such cases, we will show that accurate localization is almost impossible by exclusively dependent on a single type of measurements. However, system performance will be significantly improved by combing Doppler shift with ToA or TDoA measurements. The reason for such improvement will be unveiled theoretically and numerically.
报告人简介
Zijun Gong (Member, IEEE) received the B.Eng. and M.Eng. degrees from the Harbin Institute of Technology (HIT), Harbin, China, in 2013 and 2015, respectively, and the Ph.D. degree from the Memorial University of Newfoundland, St. John’s, NL, Canada, in 2021. From May 2021 to December 2021, he worked at the University of Waterloo as a Post-Doctoral Researcher. He is currently an Assistant Professor with the IoT Thrust in Information Hub, The Hong Kong University of Science and Technology (GZ). He is also an affiliate Assistant Professor at the ECE Department, HKUST, Hong Kong. His research interests lie in statistical signal processing and optimization, including channel estimation in massive MIMO, and millimeter wave communications, mmWave radar, radio propagation modeling, localization of WSN, and localization of underwater targets and devices. He was a recipient of the Best Paper Award at the IEEE GLOBECOM’17, Singapore, in December 2017.
04
李松泽
PhD
Assistant Professor
Internet of Things Thrust
AI Thrust
Secure Distributed Computation for Federated Learning and Blockchains
摘 要
Security and privacy issues are becoming increasingly critical to the development and deployment of modern information systems. This talk provides an overview on our recent results of developing secure, robust, and efficient distributed computation protocols, for the application of federated learning (FL) and blockchains.
Federated Learning: We expose the vulnerability of FL models via developing a novel backdoor attack that significantly improve the backdoor durability over SOTA; we develop novel secure model aggregation protocols that respectively minimizes the computation and communication overhead, while providing information-theoretic privacy for clients’ data; we also develop secure model/embedding aggregation protocols for horizontal and vertical FL scenarios, simultaneously achieving lossless performance and perfect privacy in presence of client dropouts.
Blockchains: We propose coded Merkle tree, a novel cryptographic accumulator that allows a blockchain light client to securely and efficiently verify the availability of a block; we also develop PolyShard, a novel blockchain sharding protocol that leverages coded computation to simultaneously scale the storage efficiency, throughput, and security of block verification, in presence of adaptive adversaries.
报告人简介
Dr. Songze Li is an assistant professor at the Internet of Things thrust and the artificial intelligence thrust in HKUST (GZ), and an affiliate assistant professor at CSE department in HKUST (CWB). Before joining HKUST in 2020, Dr. Li worked as a blockchain researcher at Stanford University for two years. Dr. Li received his Ph.D. degree from University of Southern California in 2018, and his B.Sc. degree from New York University in 2011, both in electrical engineering.
Dr. Li’s current research interests include AI security and privacy, secure multi-party computation, and blockchain security and scalability. Dr. Li is currently serving as the guest editor for 2 international journals on information theory and information security. He also served as a TPC member for 14 top international journals and conferences on AI and communications. Dr. Li received the Best Paper Award at NeurIPS-20 Workshop on Scalability, Privacy, and Security in Federated Learning. He was Qualcomm Innovation Fellowship finalist in 2017.
05
邢 泓
PhD
Assistant Professor
Internet of Things Thrust
Towards Communication-Efficient Over-the-Air Federated Learning: Wireless Implementations and Convergence Analysis
摘 要
With the proliferation of geo-distributed IoT devices and edge/cloud-computing applications over siloed data centres, renewed interest is gained in distributed learning, especially federated learning (FL), where multiple devices collaboratively train a shared model w/o explicit exchange of local data, thus reducing communication load while preserving users’ privacy. However, when wireless communications attempt to accommodate such model related information exchange in the last mile, communication efficiency, in the presence of trendy models with over millions of parameters, easily becomes “Achilles' heel”. To combat such issues, over-the-Air (AirComp) FL is well known to enable multiple devices to simultaneously access to the edge server over the same bandwidth resources for model aggregation. While AirComp FL does make collaborative training scalable, it encounters many challenges in implementations. In this talk, we aim for addressing some of them, namely, the mismatch between dimensions of model parameters and available wireless resources, and limitations of deploying AirComp FL in parameter-server (PS) topology. First, we introduce AirFL-Clip-Comp, which advocates dimension reduction leveraging compressive sensing and simplified power control for uncoded transmission. Next, we investigate AirFL-D2D, where novel scheduling of wireless D2D transmissions is proposed to enable analog implementation of decentralized stochastic gradient decent (DSGD). Both implementations are provided with convergence analysis, with the former highlighting its evaluation being free from realization-specific parameters, and the latter revealing the impact of connectivity and signal-to-noise ratio (SNR), respectively. Finally, theoretical insights are verified by experiments with future directions being identified.
报告人简介
Dr. Hong XING received the B.Eng. degree in Electronic Sciences and Technologies from Zhejiang University, China, in 2011, and the Ph.D. degree in Wireless Communications from King‘s College London, U.K., in 2016. Since Jan. 2022, she has been an Assistant Professor with the IoT Thrust, Information Hub, HKUST(GZ), China and an Affiliate Assistant Professor with the Dept. of ECE, HKUST, HK SAR. From Jun. 2019 to Dec. 2021, she was appointed as Research Assistant Professor with Shenzhen University, China and King’s College London, U.K.. Prior to that, she was a Post-Doctoral Research Fellow with Queen Mary University of London and King’s College London, U.K., respectively, since Feb. 2016. Her research interests include edge learning/inference, mobile-edge computing, non-orthogonal multiple access, and wireless information and power transfer. Her research output has been published in IEEE top-venue journals and conferences with two ESI highly cited paper and 1500+ citation (H-index 20, Google scholar). She received the Best 50 of IEEE Global Communications Conference (GLOBECOM) 2014.
更多学院动态
欢迎关注
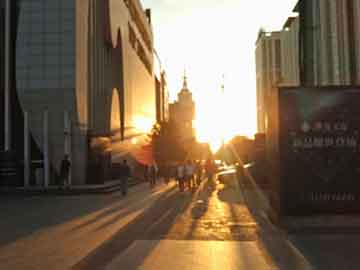
推荐站内搜索:最好用的开发软件、免费开源系统、渗透测试工具云盘下载、最新渗透测试资料、最新黑客工具下载……
还没有评论,来说两句吧...